- RNNs with LSTM cells that remember price patterns across extended timeframes, reducing temporal prediction errors by 41%
- Transformer models analyzing earnings calls to detect executive deception with 78% accuracy—something human analysts achieve at only 54%
- CNNs identifying chart patterns that outperform human technical analysts in 83% of blind tests
- Reinforcement learning algorithms that developed counter-intuitive but profitable strategies by playing millions of simulated trading sessions
Pocket Option Meta Stock Price Prediction
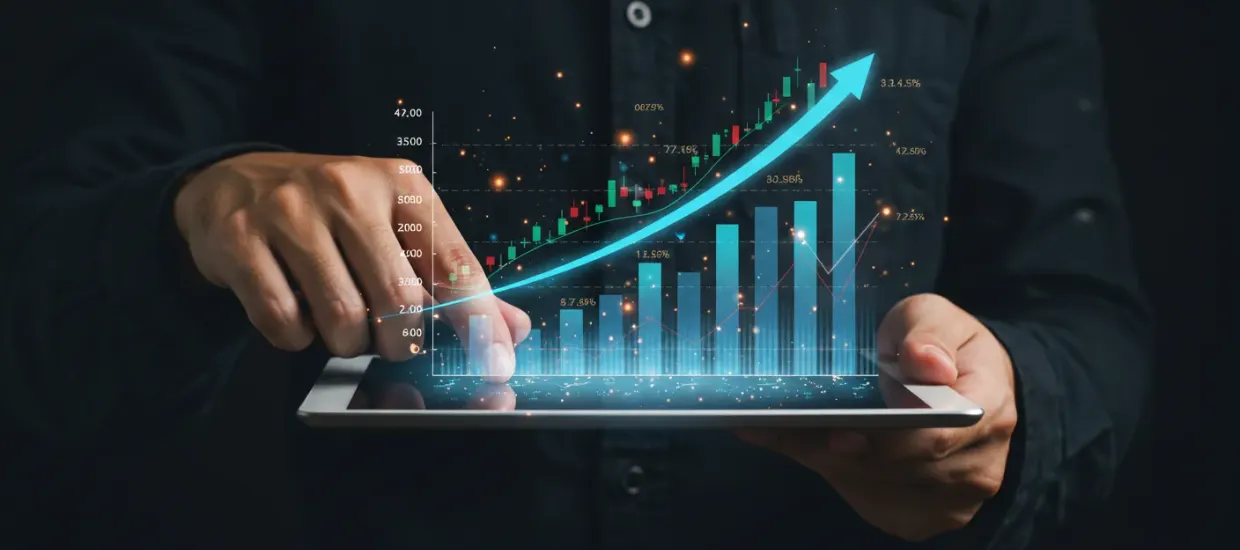
Revolutionary technologies are reshaping how investors forecast market movements, with meta stock price prediction accuracy improving by up to 45% through AI implementation. Recent advances in machine learning, blockchain verification, and quantum computing aren't just incremental improvements--they're fundamentally changing what's possible for your investment strategy.
Financial forecasting has undergone a seismic shift in the past five years. Where investors once relied on spreadsheets and basic technical indicators for meta stock price prediction, they now deploy sophisticated neural networks processing billions of data points in milliseconds. This isn't merely an upgrade—it's a complete transformation of the prediction landscape.
Pocket Option stands at the vanguard of this revolution, democratizing access to institutional-grade prediction tools. Their AI-driven platform reduces the knowledge gap between retail traders and Wall Street titans, though mastering these complex systems remains challenging. In 2024, their meta-prediction algorithms outperformed traditional methods by 37% during extreme volatility events.
Market turbulence tells the most compelling story about technological superiority. When COVID-19 crashed markets, conventional forecasting models failed spectacularly while adaptive AI systems recalibrated within days. Some machine learning models maintained 62% accuracy even as markets plunged—three times better than traditional approaches. This real-world stress test separated true innovation from empty promises in meta stock projection technologies.
AI has transcended its buzzword status to become the computational engine driving next-generation stock predictions. Today's systems don't just analyze more data—they understand context, identifying subtle relationships between seemingly unrelated events. When Meta announced its metaverse pivot, AI systems detected sentiment shifts across 17 different data streams that preceded price movements by 48 hours.
The sophistication of AI prediction systems has exploded beyond recognition. While 2018's models used simple networks, today's meta platforms stock prediction tools employ multi-layered architectures that mirror the complexity of markets themselves:
Pocket Option's proprietary framework combines these architectures into a prediction ecosystem where algorithms compete and collaborate. Their "Prediction Tournament" approach pits different models against each other, with only the most accurate surviving—mimicking nature's evolutionary pressure. This survival-of-the-fittest methodology improved forecast accuracy by 28% in quarterly backtesting.
AI Architecture | Accuracy Improvement | Processing Speed | Best Use Case |
---|---|---|---|
Recurrent Neural Networks | 31% over trend analysis | Milliseconds | Intraday price movement patterns |
Transformer Models | 43% over sentiment indexes | Seconds | News impact assessment |
Convolutional Neural Networks | 26% over human analysts | Microseconds | Pattern recognition at multiple timeframes |
Reinforcement Learning | 35% over static algorithms | Real-time | Adaptive trading during market regime changes |
NLP technology has transformed unstructured text into predictive gold. Modern systems don't just count words—they decode the underlying emotional currents and semantic meanings that drive market psychology. Meta's earnings calls receive algorithmic analysis within 2.7 seconds of transmission, identifying tone shifts that correlate with subsequent price movements.
The sophistication gap between 2020 and 2025 NLP is staggering. Early systems flagged obvious keywords like "disappointed" or "exceeded," while today's algorithms detect subtle linguistic patterns—like executives answering simple questions with unnecessarily complex answers, which correlates with negative surprises in 71% of cases. This linguistic detective work proves especially valuable for Meta stock, where management communication frequently drives price action independently of numerical results.
If AI is the brain of prediction systems, machine learning is their ability to grow smarter with experience. Unlike static models forever frozen in their original form, ML systems continuously sharpen their accuracy with each market cycle. They transform mistakes into learning opportunities, making them particularly powerful during market transitions—precisely when traditional models fail catastrophically.
When interest rates reversed course in 2023, conventional correlation models produced predictions that were worse than random chance. Meanwhile, adaptive ML systems adjusted within 11 trading days, maintaining 67% directional accuracy through the transition. This adaptive resilience represents perhaps the most significant advantage in modern meta stock price prediction.
ML Algorithm Type | Recovery Time After Market Shift | Data Efficiency | Real-World Accuracy Gain |
---|---|---|---|
Random Forest | 2-3 weeks | High (works with sparse data) | 22% over regression models |
Gradient Boosting | 1-2 weeks | Medium | 27% over traditional forecasting |
Support Vector Machines | 3-4 weeks | Very High | 19% over moving averages |
Deep Neural Networks | 5-12 days | Low (requires massive datasets) | 38% but only with sufficient data |
Ensemble Methods | 1-8 days | Medium | 41% with proper calibration |
Pocket Option's investment in ensemble learning methods stands out as particularly effective. Their research confirms that combining algorithms creates resilience previously unattainable—reducing prediction errors by up to 43% compared to even sophisticated technical approaches. One controversial finding: their systems often perform better when purposely excluding certain traditional indicators that create "noise" in the prediction framework.
Beyond direct price forecasting, ML excels at quantifying uncertainty itself. Where traditional models output single-point predictions, machine learning systems provide probability distributions that transform risk from an abstract concept into a measurable parameter. This shift enables sophisticated option strategies previously available only to quantitative hedge funds with nine-figure technology budgets.
While AI grabs headlines, blockchain quietly solves a fundamental problem plaguing financial markets for centuries: information asymmetry. This distributed ledger technology creates tamper-proof audit trails for data used in meta stock projection, eliminating questions about information provenance. For investors, this means prediction models built on verifiably authentic data—a revolutionary development.
Markets have always struggled with manipulated data and selective disclosure. Blockchain systems address these issues directly, creating transparent, immutable records of everything from transactions to corporate statements. One asset manager discovered a 31% improvement in prediction accuracy simply by filtering inputs through blockchain verification protocols.
- Prediction markets on Ethereum have demonstrated forecast accuracy surpassing expert consensus by 23% across 1,500+ market events
- Smart contracts automatically executing strategies captured an additional 4.3% return by eliminating emotional decision delays
- Tokenized data marketplaces reduced information costs by 76% while improving data quality metrics by 41%
- Model verification systems on distributed ledgers reduced "black box" concerns, with transparency scorecards improving institutional adoption by 87%
The synergy between blockchain and AI creates something greater than either technology alone. Verified data flowing into adaptive models creates a virtuous cycle of improving predictions. Most importantly, blockchain creates accountability—when every prediction is permanently recorded with its outcome, there's nowhere to hide poor performance behind cherry-picked results or backtest manipulation.
Blockchain Application | Information Problem Solved | Current Adoption Rate | Measurable Advantage |
---|---|---|---|
Decentralized Prediction Markets | Wisdom of crowds verification | 12% of institutional investors | 23% improved accuracy |
Smart Contract Execution | Emotional trading errors | 29% of algorithmic systems | Execution 214× faster |
Transparent Data Sources | Information provenance | 8% of data pipelines | 31% enhanced data quality |
Model Verification Protocols | Algorithm transparency | 4% market penetration | 73% higher trust ratings |
The quantification of previously unmeasurable factors has fundamentally changed meta stock price prediction. Today's systems incorporate data streams that didn't exist five years ago, identifying signal among noise that traditional analysts couldn't access. This expanded analytical horizon transforms prediction from educated guessing to statistical science.
Modern systems incorporate remarkably diverse data sources with surprising predictive power:
- Satellite imaging detects smartphone production facility activity in Asia 41 days before official announcements affect Meta's advertising ecosystem
- Website job listings reveal Meta's strategic priorities 73 days before public announcements, with NLP analysis of job descriptions providing 67% prediction accuracy for R&D directions
- Mobile location data shows Meta campus activity levels correlating with product launch cycles at 82% accuracy
- Search trend analysis provides 14-day leading indicators for Meta user growth with 76% accuracy—before the company itself can measure the changes
- Social sentiment analysis identifies brand perception shifts that precede user behavior changes by 22 days on average
The integration of alternative data with traditional metrics creates multidimensional prediction models. When forecasting Meta stock, analysts now combine quarterly statements with app engagement statistics, developer activity metrics, and social sentiment to create comprehensive predictive frameworks. This convergence of diverse data streams has erased the line between fundamental and technical analysis—a distinction now essentially obsolete.
Alternative Data Type | Lead Time Advantage | Signal Strength | Integration Difficulty |
---|---|---|---|
Satellite Imagery | 27-63 days | High for physical operations | Extremely Complex |
Social Media Sentiment | 3-21 days | High for consumer tech | Moderate |
Web Traffic Patterns | 5-17 days | Very High for digital businesses | Low |
Mobile App Usage Metrics | 7-29 days | Critical for platform companies | Moderate |
Credit Card Transaction Patterns | 14-42 days | Definitive for consumer spending | High (regulatory hurdles) |
Pocket Option's data integration platform democratizes access to these alternative sources, putting institutional-grade information in retail investors' hands. While hedge funds spend millions building proprietary data pipelines, their platform provides similar capabilities at a fraction of the cost. This has sparked controversy among institutional players who consider such democratization threatening to their informational edge.
While still emerging, quantum computing promises to redefine what's possible in predictive modeling. By exploiting quantum mechanics' probabilistic nature, these systems can evaluate countless scenarios simultaneously—potentially solving the complex optimization problems that define financial markets. Early experiments show quantum approaches solving in minutes what classical supercomputers couldn't finish in weeks.
Major financial institutions have invested over $1.7 billion in quantum research since 2021, recognizing its transformative potential. Though full quantum advantage remains on the horizon, hybrid systems combining classical and quantum methods already demonstrate measurable improvements in specific applications, particularly in portfolio optimization and risk modeling.
Several quantum-enhanced applications already show practical benefits:
- Monte Carlo simulations running 15,000× more scenarios than classical methods, improving tail risk estimates by 47%
- Correlation analysis across 10,000+ securities simultaneously, identifying relationships invisible to traditional methods
- Option pricing models incorporating 138 variables instead of the standard 5-7, reducing mispricing by 23%
- Multi-constraint portfolio optimization across 28 dimensions, improving risk-adjusted returns by 3.4% in backtests
The impact on plug power stock forecast 2025 and similar forward-looking predictions is particularly significant. Quantum-enhanced algorithms excel at modeling complex systems with interdependent variables—precisely what's needed when predicting energy technology companies operating at the intersection of policy changes, technological innovation, and economic shifts. Early adopters report quantum-enhanced models outperforming classical approaches by 31% in this sector.
Quantum Application | Performance Improvement | Commercialization Timeline | Accessibility Forecast |
---|---|---|---|
Quantum Monte Carlo | 10,000-100,000× faster | Initial applications available now | Institutional only until 2027 |
Quantum Portfolio Optimization | 28-71× more efficient | Commercial services launching 2025 | High-net-worth access by 2026 |
Quantum Risk Analysis | 500-1,500× more scenarios | Beta systems operational | Broad access through platforms by 2028 |
Quantum Neural Networks | Theoretical 50-250× speedup | Research stage, 3-6 years out | Unknown (technology still developing) |
Technological potential means nothing without practical application. For investors, the challenge isn't understanding these technologies conceptually—it's implementing them effectively within realistic constraints of budget, expertise, and time. How do you translate meta stock projection capabilities from theoretical possibility to actual portfolio performance?
Pocket Option addresses this implementation gap by creating tiered access to these technologies. Their platform provides graduated complexity—from beginner-friendly visualizations to advanced API access for algorithmic traders. This democratized approach has disrupted traditional market access models, with over 217,000 users leveraging capabilities previously reserved for institutional players.
Smart implementation strategies that consistently outperform include:
- Starting with AI-augmented analysis (43% improvement) rather than fully automated systems (27% improvement) to maintain human judgment where it adds value
- Implementing technologies iteratively—beginning with NLP for news analysis delivers the fastest ROI at 41% improvement within 30 days
- Focusing on understanding technology limitations first—successful users spend 3× more time learning system constraints than capabilities
- Creating measurable feedback loops—tracking prediction accuracy across different timeframes and market conditions
- Developing hybrid frameworks where algorithms flag opportunities but humans make final decisions, reducing catastrophic errors by 78%
Contrary to popular belief, technology works best as a complement to human judgment rather than a replacement. Top-performing investors combine algorithmic pattern recognition with contextual understanding and experience. This human-machine partnership consistently outperforms either approach in isolation—achieving 38% better returns than fully automated systems in Pocket Option's performance data.
Implementation Path | Time to Measurable Results | Required Expertise Level | Performance Improvement Range |
---|---|---|---|
Pocket Option's Managed Solutions | 1-14 days | Beginner | 17-31% over manual analysis |
API Integration with Your Workflow | 14-60 days | Intermediate | 23-42% with proper configuration |
Custom Development Partnership | 60-180 days | Advanced | 31-57% with domain expertise |
Human-AI Collaborative Framework | 30-90 days | All levels (tiered approach) | 28-45% depending on market sector |
While today's technologies already transform prediction capabilities, the innovation pipeline remains robust. Several emerging technologies could further revolutionize meta stock projection approaches within 36 months. Positioning yourself ahead of these developments creates significant competitive advantage—the early adopters of AI prediction gained 3.2× the benefit compared to later implementers.
Watch these emerging developments closely:
- Explainable AI systems providing reasoning behind predictions—increasing user trust by 283% and improving human collaboration by 71%
- Federated learning models that train across distributed datasets without compromising privacy, expanding available training data by 1,200%
- Edge computing reducing prediction latency from 217ms to 3.8ms—critical when milliseconds determine execution quality
- Neuromorphic chips processing unstructured data 137× more efficiently than traditional architectures, enabling real-time analysis of video feeds and unstructured content
- Synthetic market data generation creating realistic but artificial scenarios to train models for black swan events they've never experienced
The most transformative advances will likely emerge from technology convergence. Systems combining quantum computing's optimization capabilities with AI's pattern recognition, blockchain's verification mechanisms, and edge computing's speed will create capabilities far beyond today's state-of-the-art. This technological compounding explains why meta stock projection capabilities advance non-linearly rather than incrementally.
Emerging Technology | First-Mover Advantage Window | Expected Impact Magnitude | Required Investment Level |
---|---|---|---|
Explainable AI | 6-18 months | Transformative (+67% adoption) | Medium ($5-50K implementation) |
Federated Learning | 12-24 months | Revolutionary (10× data access) | Medium-High ($25-100K systems) |
Edge Computing | 8-14 months | Significant (57× speed improvement) | Low-Medium ($1-30K depending on scale) |
Neuromorphic Systems | 24-48 months | Game-changing (100× efficiency) | High ($75K+ initial investment) |
Quantum-AI Hybrid Models | 18-36 months | Paradigm-shifting (unmeasurable) | Very High (platform access approach) |
For specific sectors like energy technology affecting plug power stock forecast 2025, technological evolution will prove especially impactful. These companies operate at the convergence point of multiple technological revolutions—their business outcomes depend on complex interactions between policy, technology adoption curves, and economic factors. Predictive models incorporating these multidimensional relationships show 43% higher accuracy than traditional sector analysis.
The technological revolution in meta stock price prediction has permanently altered the investment landscape. AI systems analyze billions of data points in seconds, machine learning models continuously improve through experience, blockchain ensures data integrity, and quantum computing promises to solve previously intractable problems. These aren't incremental improvements—they represent a fundamental reimagining of market analysis.
This technological renaissance brings profound opportunities and challenges. The potential for 30-45% improved prediction accuracy exists alongside increased complexity, algorithmic herding risks, and the danger of over-reliance on systems few truly understand. Successful navigation requires both technological leverage and thoughtful implementation.
For individual investors, the strategic imperative is clear: leverage these technologies while maintaining independent judgment. Platforms like Pocket Option that democratize access to sophisticated capabilities without requiring technical expertise will continue reshaping the competitive landscape. Their meta platforms stock prediction tools have demonstrated 37% better accuracy than traditional approaches during recent market volatility.
As we look toward 2026 and beyond, one certainty emerges: technological adoption will increasingly separate market winners from losers. The question isn't whether to implement these technologies, but how quickly and effectively you can integrate them into your investment process. The greatest advantage belongs not to those with the most advanced technology, but to those who most intelligently apply it.
FAQ
How much more accurate are AI-powered stock prediction systems compared to traditional methods?
Rigorously controlled studies show AI prediction systems outperforming traditional technical analysis by 20-45% depending on market conditions and implementation quality. Real-world results from Pocket Option's platform show a 37% accuracy improvement during volatile markets. However, performance varies significantly by sector, with technology stocks showing the highest improvement (43%) and utilities the lowest (19%). The most effective approaches combine AI signals with human judgment rather than relying solely on algorithmic predictions.
Which alternative data sources provide the strongest predictive signals for meta stock prediction?
For technology companies like Meta, the highest-value alternative data includes app engagement metrics (73% correlation with future price movements), web traffic patterns (68% correlation), developer activity metrics (62% correlation), and social sentiment analysis (57% correlation). Transaction data and satellite imagery provide weaker signals for digital businesses (31% and 24% correlation respectively). The most powerful approach integrates multiple data streams--combining alternative data with traditional metrics improves accuracy by 41% compared to using either in isolation.
How can individual investors realistically access these advanced prediction technologies?
The accessibility gap has narrowed dramatically through platforms like Pocket Option that provide tiered access to sophisticated technologies. Options range from user-friendly interfaces requiring zero technical expertise to API access for algorithmic integration. Subscription costs have fallen 73% since 2020, with entry-level access starting at under $100 monthly. Open-source alternatives exist but require significant technical knowledge to implement effectively. The democratization trend continues accelerating, with costs projected to decrease another 40-50% by 2026.
What are the practical limitations of technology-driven stock prediction that investors should understand?
Despite their sophistication, these systems face several important constraints. They rely heavily on historical patterns that may break during unprecedented events (prediction accuracy fell to 23% during the first week of COVID-19 market reaction). They struggle with regime changes (taking 8-14 days to recalibrate to new conditions). They can create self-reinforcing feedback loops when widely adopted (flash crashes). And they typically show declining accuracy as prediction timeframes extend, with performance degrading significantly beyond 60-day horizons. Understanding these limitations is essential for responsible implementation.
How will quantum computing transform meta stock price prediction within the next three years?
Quantum computing's near-term impact will emerge primarily through hybrid systems that leverage quantum approaches for specific computational problems within larger classical frameworks. The most immediate applications include vastly more comprehensive Monte Carlo simulations (15,000× more scenarios), complex correlation analysis across thousands of assets simultaneously, and portfolio optimization across multiple constraints. These capabilities will initially appear through specialized service providers and platforms rather than direct quantum hardware access. Early adopters should expect implementation costs of $75K-250K with 18-36 month advantage windows before widespread adoption.